Nyse Article About Against Social Media
Abstruse
Since the 2019 novel Coronavirus disease (COVID-19) spread across the world, risks brought by the pandemic set up in and stock markets tumbled worldwide. Amongst the dour economic outlook, investors' concerns over the pandemic spread rapidly through social media just wore out shortly. Similarly, the crash only caused a relatively brusque-lived bear market, which bottomed out and recovered quickly. Meanwhile, engineering science stocks have grabbed the spotlight equally the digitally avant-garde sectors seemed to show resilience in this Coronavirus-plagued marketplace. This newspaper aims to examine market sentiments using social media to predict the stock market performance before, during and afterward the March 2020 stock market crash. In addition, using the Organisation for Economic Co-functioning and Evolution Taxonomy of Sectoral Digital-intensity Framework, we identified market sectors that take outperformed others as the market sentiment was impacted by the unfolding of the pandemic. The daily stock performance of a usable sample of 1619 firms from 34 sectors was first examined via a combination of hierarchical clustering and shape-based distance measure. This was then tested confronting a fourth dimension series of daily price changes through augmented vector auto-regression. Results testify that market sentiments towards the pandemic have significantly impacted the price differences. More interestingly, the stock performance across sectors is characterized by the level of digital intensity, with the well-nigh digitally avant-garde sectors demonstrating resilience confronting negative market sentiments on the pandemic. This inquiry is among the showtime to demonstrate how digital intensity mitigates the negative consequence of a crisis on stock market place performance.
Introduction
The outbreak of 2019 novel Coronavirus disease (COVID-nineteen) has brought widespread risks and investor concerns worldwide, with the stock prices in all major stock markets seeing sudden and unprecedented autumn. The Coronavirus stock market place crash was among the fastest falls in financial history [1]. Among the bleak economic outlook, investors' concerns over the pandemic seemed to spread rapidly through social media but wore out shortly afterwards that. Social media data measure people's attention on unexpected incidents and can serve as a timely indicator that drives investment dynamics. For example, salient patterns exhibited in social media accept been employed as an unconventional source of strategic information to predict stock market movements [two]. In fact, like the sudden surge and decline in social media postings on the pandemic, the crash but caused a relatively short-lived deport market, which bottomed out and recovered speedily.
Meanwhile, many countries have imposed lockdown measures in attempts to slow the spread of the life-threatening virus, from mandatory geographic quarantines, not-mandatory recommendations to working from dwelling house, closures of certain types of business entities, or bans on mass events and gatherings. These measures have brought those that are categorized equally 'not-essential' brick-and-mortar business organisation activities shut to consummate standstill. Even so, reports have suggested that even as most corporeal business activities halted, merchandise and consumption continued to grow online as the virus peaked [3]. This is also well reflected in the financial markets—technology stocks have grabbed the spotlight every bit the digitally intensive sectors seemed to show resilience in this Coronavirus-plagued market. Changes in consumer habits—an accelerated embrace of east-commerce, dwelling house amusement and working from home [four]—have strengthened the already dominant positions of a few mega-cap technology companies, such as Amazon.com, PayPal and Alphabet, Google'due south parent company[5].
Data science, coupled with computational biological science, is helping in myriad ways with applications including epidemiology, drug discovery, and molecular pattern for diagnostic and therapeutic purposes [6,vii,eight]. A number of data driven models, mathematical models, and predictive models have been developed for COVID-19 [ix,10,11,12,13]. This paper aims to apply information science methods to predict the stock marketplace performance using social media data earlier, during and afterwards the March 2020 stock market crash. Specifically, using the Organisation for Economic Co-functioning and Development (OECD) Taxonomy of Sectoral Digital-intensity Framework [14], nosotros model market place sentiments using cumulative Twitter postings on COVID-19 to predict the stock market performance and investigate what are the sectors that have outperformed others as market place sentiments are impacted by the unfolding pandemic. In particular, nosotros are interested to find out how digital intensity moderates the effect of market sentiments on firms' stock prices, specially due to largescale unanticipated events such every bit a pandemic.
This research contributes to the existing literature by examining the influence of market sentiments associated with COVID-19, every bit derived using cumulative social media postings, upon the stock marketplace performance. While previous enquiry has considered the employ of social media to meliorate stock performance forecasting [15, 16], this research is amidst the first few to demonstrate how digital intensity could mitigate the negative result of market sentiments on stock market operation in a time of crisis.
The rest of the paper is organized as follows. The next department introduces the extant literature roofing three fields of research: the touch on of largescale unanticipated incidents on stock operation; the relationships between social media and the stock markets; and the sectoral digital intensity. This is then followed by a section that depicts the empirical study, detailing the research method and data analysis. The discussion department features the implications drawn from the fundamental findings to both research and practise.
Literature Review
This research aims to investigate what are the sectors that accept a competitive advantage despite the adverse issue of the pandemic. The subsequent sub-section looks at social media and financial markets, reviewing the literature on how social media data have been employed in examining investors' sentiments and stock market performance. Furthermore, because that digitally advanced firms performed ostensibly ameliorate in the financial markets, the OECD taxonomy of sectors past digital-intensity [14], which is adopted as the framework for classifying the sectors in the present report, is then introduced.
Result of Largescale Unanticipated Events on Stock Markets
According to the Efficient Market Hypothesis (EMH), the aggregate decisions of all participants in the market should accurately reflect the value of public companies and their common shares at any given moment of time. Yet, empirical prove has shown that, in violation of EMH, people tend to "overreact" to unanticipated and dramatic news events [17, 18]. Over-reaction may occur in the short run when investors plow extremely pessimistic during downturns, or place too much emphasis on recent incidents while discounting historical data, which results in prices falling excessively on bad news [18].
In the current research, the COVID-nineteen pandemic is conceptualized as an case of large-scale unanticipated adverse events. In the past, diverse studies take examined the impact of disasters or major incidents on stock markets. For instance, Barrett et al. [19] studied the effects of aviation accidents on stock returns in 1987; Shelor et al. [20] and Lee et al. [21] analysed the effects of natural disasters, such every bit hurricanes and earthquakes, on the stock markets. Cam and Ramiah [22] studied how investors react in the presence of unique large-scale disasters, such every bit the ix/eleven terrorist attacks. Their results show considerable stock market overreactions, which are in understanding with an availability bias acknowledged in the behavioural finance literature, driven by the unprecedented loss and the successive high media attention these events typically receive [23].
Like other types of catastrophic events, COVID-19 is characterized by extreme uncertainty, whose transmission characteristics and consequences to human bodies were largely unknown. The rapid increment in new cases and expiry tolls take a significant emotional and material touch worldwide. The virus has certainly adjusted the investor perception on the micro and macroeconomic outlook, resulting in a 'market-wide shock'. In fact, the touch of COVID-19 on the stock market is more meaning than previous disasters due to its fast transmission across the earth and information technology is expected to co-be with humans over the long-term [24].
In the by, there have been many epidemics that impacted stock markets worldwide, such as Influenza, Ebola, and Severe Acute Respiratory Syndrome (SARS). All of these events have caused extensive impact on the financial markets. For the United States, a higher incidence of flu is associated with decreased trading, decreased volatility, decreased returns, and higher bid-ask spreads [25]. SARS infected more than 8000 people in 2003 and shaved 12.8% off the Standard & Poor (S&P) 500 over 38 trading days; Avian Influenza in 2004, Middle Due east Respiratory Syndrome (MERS) in 2012 and Ebola in 2013–2014 resulted in 5.8 to 7.three% drop to the South&P 500 over different lengths of fourth dimension. Similarly, the market suffered a near 12.9% pullback in a span of 66 trading days due to the outbreak of Zika virus between 2015 and 2016 [26]. Nonetheless, none of the preceding epidemics were comparable to COVID-19 in terms of its number of cases infected and devastation to the economic system and the financial markets. In order to end the transmission, countries have close downwards their business and people are advised to stay home. As a outcome, unemployment rates have increased; tourism and supply bondage around the globe have been disrupted [27]. Khurram, Liu and Hayfa [24] argue that COVID-19 has paused the economic cycle throughout the world, and that the wellness crunch has successfully induced the global financial crunch. Since the outbreak of COVID-xix, major financial markets, such as the Southward&P 500, National Clan of Securities Dealers Automatic Quotations Organization (NASDAQ) 100, and Nikkei 225, have been confronted with a severe collision of nearly xxx% in marketplace values. Markets are close to collapse equally they were during the Global Financial Crisis (GFC) between 2007 and 2009 [28], but more perilous [29]. In addition, the financial volatility alphabetize (VIX), also known every bit "Fright approximate," has moved to the highest level, while the 10-twelvemonth treasury yield index in the United states of america has dropped to record low figures [27]. According to an cess past the Asian Development Bank (ADB), the global toll of COVID-19 could be $4.1 trillion.
While the economies are being hit hard, nosotros observe an most Five-shaped recovery in several stock markets, despite the international gloomy economic outlook. There are many discussions on the possible reason behind the rapid rebound, such as fiscal stimulus from the Fed and Congress, expectations of a strong economic recovery and major engineering stocks that help bulldoze the gains [thirty, 31]. This newspaper posits that the stock market rebound is partly attributed to the fading worries virtually the virus. The measures taken by nations effectually the world to manage COVID-xix—restricting travel, shuttering nonessential businesses and mandatory social distancing policies—are having obvious impacts on slowing contagion. Coronavirus complacency arrives after overblown fears fuelled by media, which seem to contribute to the volatility of the stock marketplace.
Social Media and Stock Markets
Most conventional research that examines the touch on of largescale unanticipated events on stocks accept mainly used intervention assay [32] or event studies [33]. These incidents are typically operationalized every bit dummy variables, which are not able to directly assess the public attending to outlying events, nor quantify such effects. Some exceptions include Liu et al.'s [34] written report on aviation accidents and Ding et al.'s [35] enquiry on the bear upon of COVID-nineteen. Both Liu et al. [34] and Ding et al. [35] make up one's mind market sentiments towards critical events using Search Indices, and assess their effects on stock prices.
Stock prices are influenced by a multifariousness of cardinal factors. Investor sentiments play an important role in shaping the stock market [36]. Numerous professional person and amateur analysts and investors use Twitter to mail news manufactures and opinions, often more frequently than the mainstream news media [16]. As a meaningful aqueduct for users to share information, social media have been integrated into many aspects of decision-making processes in our daily lives [37], including investment decisions [xv]. Social media data reveal significant public involvement, most in existent time. As such, it serves every bit a convenient and advisable source to measure out marketplace sentiments.
Several extant studies have shown that social media data measure out people'southward attention and sentiments and provide timely feedback on investment dynamics. Bollen et al. [2] reveal that Twitter feeds are correlated to the value of the Dow Jones Industrial Average (DJIA) over fourth dimension. Their work is in line with the findings of Gilbert and Karahalios [38], who construct public anxiety indices from online comments to estimate moves of the South&P 500. Several other of import studies take also shed light on the various aspects of financial market performance in relation to social media. For example, Sprenger et al. [16] deploy machine learning algorithms to construct a unlike bullishness index, which is predictive of stock returns days later. Smailovic, Grcar, Lavrac, and Znidarsic [39] observe sentiment (i.e., positive emotion) in Twitter postings to exist predictive of stock returns. Saini and Sharma conduct a comparative analysis of diverse prediction techniques deployed to predict stock price using public sentiments from social media and other news sources [xl]. These results confirmed the feasibility of using social media as a proxy for market sentiments.
Sul et al., [15] analyse the cumulative sentiments (positive and negative) in tweets on S&P 500 firms and model them against the stock returns of those firms. Cumulative posts that capture the collective interest of investors are an appropriate reflection of market sentiments and useful indicators for predicting investment decision making [15]. This report builds upon Sul et al. [fifteen] in applying cumulative social media feeds as an indication of commonage attention towards a largescale unanticipated incident, i.e. COVID-xix, to study their bear on on the stock performance across sectors.
Digital Intensity of Sectors
Despite the spread of COVID-19 resulting in the stalling of brick-and-mortar business activities, reports have shown that COVID-19 is accelerating the rising of the digital trade. Digitally-enabled companies are thriving in a time of crisis [3, 41]. Firms that have undergone digitalisation are more capable to maintain some caste of business organisation functioning and revenue stream amidst the pandemic. Changes are affecting multiple aspects (due east.g. product, procedure and business model innovation) and stages of innovation (e.g. enquiry, development, commercialisation), the prevalence of which may differ across sectors [42].
Recent OECD piece of work [14] benchmarks sectors in accordance to their level of digital intensity. The OECD'due south Taxonomy of Digital Intensive Sectors is one of the virtually established sectorial digitalization frameworks [42, 43]. This framework provides a systematic overview of digitalisation and its various manifestations: its technological indicators consist of Information and Communications Technology (ICT) equipment and software investment relative to full fixed investment; Purchases of ICT intermediate goods and services relative to output; Stock of robots per employee; Number of ICT specialists over total employment (as well referred to as "ICT-specialist intensity"); and Propensity to engage in east-commerce sales. The indicators considered highlight how the degree of digitalisation in sectors is shaped by firms' investments in various "digital" assets, the approach to interact with markets, the (type of) human capital and skills required, and the way production is operationalized.
There are 36 sectors in the OECD Structural Analysis (STAN) database, ranked by their intensity in the above-mentioned dimensions. For each indicator, cantankerous-country averages are calculated at the sector level and used to benchmark each sector relative to all the others. Table 1 displays an overall summary of the sectors past quartile of digital intensity. Specifically, the taxonomy lists sectors according to their relative position in the overall economic system'due south ranking and categorizes them into "high", "medium–loftier", "medium–low" and "low" digital intensity, depending on whether sectors appear in the top 25% (or quartile, denoted as "high"), in the lesser 25% ("low"), or in between the two.
Every bit illustrated in Table 1, some sectors lag in the degree of digitalisation, such as agriculture, mining, and real estate. By contrast, the technology sector, media, financial services, and professional services are ahead of the bend. The uneven development of digitalisation matters as it is creating a new digital divide between the digital "haves" and "accept-mores" across sectors. Firms with advanced digital assets and capabilities have been found to enjoy faster growth in revenue and profit margin and generate higher return to shareholders [14].
Digital transformation is a multifaceted phenomenon that has profound impact. Technologies accept always served equally key resources in treatment bug with regards to complex product-service systems [44]. For manufacturing companies, a service-oriented transformation enabled past technological revolution is accounted to be important by both trade [45] and bookish literature [46]. Technologies such every bit the Net of things and bogus intelligence offer new pathways to innovative business models, transforming traditional manufacturing to smart manufacturing where the operations require minimal human intervention[47].
In a post-COVID-19 'new normal', it is posited that in enhancing societal and economical resilience, digital connectivity has emerged every bit a crucial alternative to the physical equivalent [48]. It is observed that the stock price of Zoom Video Communications, Inc., a web conferencing platform provider, has risen from United states of america$70 in early on Jan 2020 to Us$150 at the terminate of March 2020 among the market crash. The "GAFAM" stocks—Google; Apple tree; Facebook; Amazon; and Microsoft—have significantly outperformed broader market indices considering of travel restrictions and social distancing orders [five]. Every bit many companies plunge into the financial unknown, sectors with a pre-existing digital ecosystem instil investors' confidence in the companies and their stocks [3, 49]. Thus, sectors that have embarked on digital transformation, the digital "have-mores", are more resilient to the adverse result of market sentiments from COVID-19, while other sectors that are succumbing with total standstill are amongst the nearly negatively afflicted.
Empirical Study
Inquiry Design
To differentiate the sectors that have performed relatively well from those that have been more than heavily impacted past the unfolding of the pandemic, we carry out an empirical study using data from daily cumulative Twitter feeds on COVID-nineteen and stock prices. The OECD Taxonomy of Sectoral Digital-intensity Framework [xiv] is referenced to classify the sectors.
The written report comprises ii phases. The first Phase used hierarchical clustering and shape-based altitude (SBD) to validate the categorisation of firms specified in the OECD Sectoral Digital-intensity Framework, extending the approaches by Sardá-Espinosa [50] and Paparrizos and Gravano [51]. The assay identified two distinct clusters, i.e., a "sensitive" cluster and a "resilient" cluster. The second Phase modelled daily cumulative Twitter feeds on COVID-19 and stock cost changes in an augmented vector machine-regression (VAR) [52]. The assay compared the stock performance of firms across the two levels of digital intensity, i.east., low/medium, and loftier, co-ordinate to the OECD Sectoral Digital-intensity Framework. The empirical investigation in the two phases congenital upon each other, supporting the notion that market sentiments towards COVID-19 as reflected in Twitter feeds affect the stock prices.
Data Sources and Assay
Using data from FactSet, nosotros selected close to 2000 firms listed on the NASDAQ according to the sectoral information in 2020. As one of the world'southward largest stock exchange based on market capitalization, NASDAQ represents ane of the most extensively studied stock exchange database amidst the academic customs [53, 54]. Information drove from such a popular platform facilitates gleaning findings that would concord both bookish and practical significance. Sectors are selected according to the OECD Taxonomy of Sectoral Digital-intensity Framework [fourteen]. The sample comprised four categories of firms: "high", "medium–high", "medium–depression" and "low" digital intensity, depending on whether sectors appear in the pinnacle 25% (or quartile, denoted every bit "high"), in the bottom 25% ("depression"), or in between the 2.
From the initial sample, all entries with missing values were eliminated. Pharmaceutical and biopharmaceutical sectors are playing an essential role at the front end lines against the virus. While global stock markets take a COVID-19 hit, pharmaceutical stocks are generally outperforming the others, with increased investments in the race to develop therapeutics and vaccines. Manufacturers of diagnostic kits, sanitizers and surgical masks are all ramping up product to see unprecedented need. Equally the present study mainly focuses on how digitally advanced sectors show resilience confronting negative market sentiments under crunch, firms belonging to the pharmaceutical and biopharmaceutical sectors were removed from the sample to avoid confounding effects. Additionally, we also removed hotels, resorts and cruise lines from the sample. Every bit one of the hardest-striking industries, these sectors were largely affected past travel restrictions, social distancing and lockdowns, which are non relevant to the research focus of digitalisation. The terminal dataset consists of 1619 firms from 34 sectors.
Although these stocks in the sample cover only 31% of all stocks on the NASDAQ, they correspond an economically significant segment of the total market place as they account for close to 40% of total NASDAQ marketplace capitalization in the first half of 2020. The average market capitalization of the sampled stocks is United states$eight,540 million, with a median value of US$643 million. The sampled time flow (all trading days between early January to early on May) allows a reasonable amount of time lag for the COVID-19 outbreak to generate public attending and stir market place response. Table ii summarizes the descriptive statistics of the firms in each sector. From the column of boilerplate daily price changes during the sample fourth dimension flow, some sectors (e.grand., agronomical commodity, apparel/footwear retail, structure, etc.) exhibit negative cost movement, whereas some sectors such as Cyberspace retail and telecommunication that are likely to exist resilient during the pandemic showroom positive price change. Additionally, we examine the stock price trends of the top companies in two sampled industries (i.e., chemical products and Internet retail) before, during and after the March 2020 stock market crash (come across Fig. 1). A mixed effect of the COVID-19 outbreak is observed in the chemic products sectors. In contrast, industries like Internet retail provide a silver lining amid the gloomy stock marketplace with a stronger turn-effectually than other sectors. Stocks exhibit different degrees of rebound from the pandemic crash. In Phase 1, nosotros intend to group together firms of like performance in Coronavirus-plagued market and similar degree of rebound following the marketplace crash.
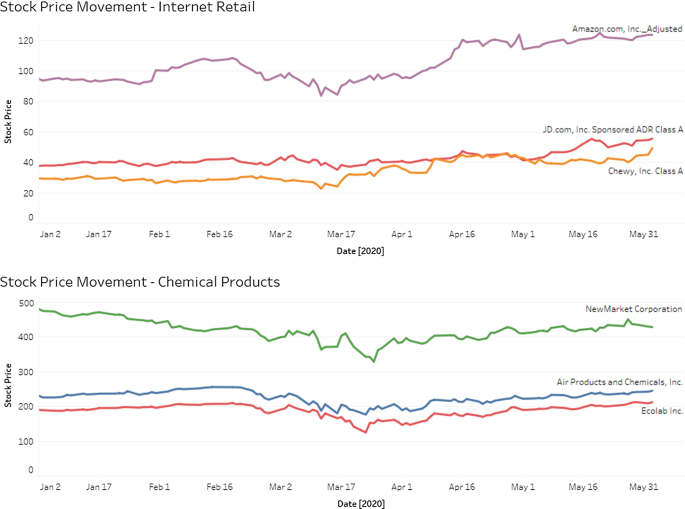
Stock Price Movement of Representative Industries. The stock prices of Amazon.com.Inc were scaled downwardly (i.east., bodily stock price/xx) to fit into a comparable view with other companies in the Internet retail sector
In Phase 2, the daily cumulative Twitter postings on COVID-nineteen are collected from Tweet Binder [55]. Between mid-January 2020 to early on May, in that location were 628,809,016 tweets generated on COVID-19 on Twitter [55], which captured the evolution of several keywords: #covid19 OR #Coronavirus OR Coronavirus OR #covid-19. In mid-March, Twitter showed an unprecedented increment in tweets related to the Coronavirus in a very brusque span of time (see Fig. 2). Cumulative Twitter postings have been used as a source of data in several studies [fifteen], yet how such social media behaviours evolve during the course of a pandemic has not been extensively studied hitherto. Nosotros posit that such daily cumulative data are a timely reflection of the market attention on the catastrophic crunch. This inquiry is the showtime to employ social media feeds to examine how critical incidents influence stock prices of firms beyond different sectors. Such data not only offer insights into investors' collective attention under unexpected incidents, but besides reverberate the changes over time.
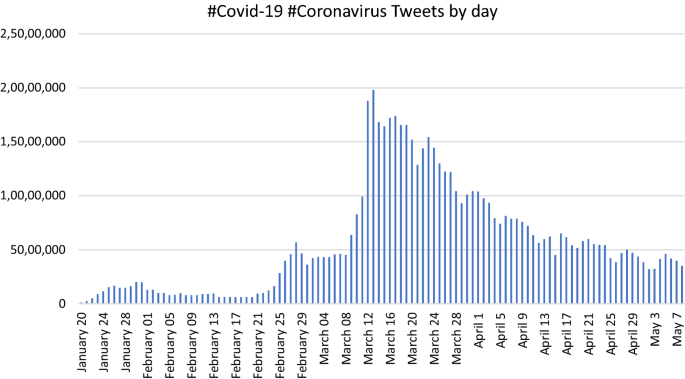
Source: Tweet Folder [46]
Twitter feeds related to COVID-19. The streamer captures the daily cumulative tweet counts by #covid19 OR #Coronavirus OR Coronavirus OR #covid-19 to reverberate the evolution of tag words.
Results
Stage 1 Results
Instead of comparing static descriptive indicators of firms, this report employs clustering methodology on dynamic stock time series from Jan 2020 to early June 2020 [56]. The option of time-series clustering approaches depends on the elements of (dis)similarity or altitude measures, clustering algorithms and evaluation [56, 57]. Liao [58] summarizes that a majority of time-series clustering methods are adapted from static clustering algorithms with alteration of similarity or image extraction function, or transformation that converts dynamic serial to obtain static features. Most time series clustering approaches are based on g-means and hierarchical clustering with different specifications of (dis)similarity measures suitable for the problems. Still, k-means algorithm variations (due east.thousand., fuzzy c-means) are more compatible to analyse time series of equal length [56, 58, 59]. Hierarchical clustering is more applicable to our dataset of daily market capitalization every bit non all the firms trade every day. It also requires a distance measure such equally Dynamic Fourth dimension Warping (DTW) distance to generate dissimilarity matrices [51].
Hierarchical clustering method groups objects into tree-based clusters including agglomerative method and divisive approach [threescore]. The agglomerative tree starts with single object clusters and iteratively groups them into larger clusters past dissimilarity measures. All objects volition merge into one cluster unless cease criteria are defined. It volition end if all objects are grouped into one cluster or certain criteria are satisfied. For example, the agglomerative tree continues growing until it gets to the maximum number of clusters. The divisive tree starts with 1 cluster having all objects and iteratively splitting the cluster until all objects reach single object clusters. The visualized result of hierarchical clustering depicts a binary tree which is constructed by nodes and their children nodes. The children nodes under one parent node are more like each other compared to the children nodes that belong to some other parent node. Similarity between clusters is defined in two ways, namely single linkage and consummate linkage. The old takes the similarity of the closest pair, whereas the latter takes the similarity of the furthest pair [60]. DTW distance is a popular choice in the context of time-serial clustering [50, 56]. DTW compares time series where the timing or the tempo of the variations may vary between the series [61]. However, information technology is a computationally expensive option which takes multiple approaches of optimization to meliorate its time and memory efficiency [52].
Nosotros follow two steps to conduct hierarchical clustering using DTW distance [61]. The first step is to calculate the distance betwixt time serial using the DTW method. It is followed by calculating hierarchical cluster analysis over these dissimilarities. To calculate a DTW distance, considering any two time series to compare, \(X\left( {x_{ane} ,x_{2} , \ldots , x_{n} } \right)\) and \(Y\left( {y_{one} ,y_{2} , \ldots , y_{grand} } \correct)\), the commencement step is to compute a local toll matrix (lcm), which has all pairwise distances with \(n \times one thousand\) dimensions. \(p\) corresponds to the \(l_{p}\) norm that defines the altitude measures used to construct the lcm matrix. As the second step, an optimum path denoted as Ď• = {(1, i),…,(n, m)} is searched to minimize the alignment between X and Y past iteratively looping through the lcm from lcm(1, one) to lcm(n, m). For each stride, the management would be identified when the least cost increased under the chosen constraints [62]. The terminal distance \(DTW_{p} \left( {x, y} \right)\) is computed in Eq. (i) using m Ď• the per-step weighting coefficient and Thousand Ď• the corresponding normalization abiding.
$$DTW_{p} \left( {x,y} \correct) = \left( {\mathop \sum \limits_{{}}^{{}} \frac{{m_{\phi } lcm\left( k \correct)^{p} }}{{M_{\phi } }}} \right)^{1/p} , \quad \forall {\text{k }} \in { }\phi { }$$
(1)
We apply the DTW algorithm to all stock prices over the sample time menstruum which are represented as numeric matrices and interpreted row-wise. The primary outputs of the DTW algorithm are the minimum global distance between each pair of stock price time series. This distance matrix acts equally inputs to enter hierarchical clustering. The hierarchical DTW clustering is conducted based on z-scores normalization with shape-based centroid. Some other important decision is to cull the optimal number of clusters when using hierarchical clustering, however, this determination could be subjective. The recommended evaluation methods of clustering performance are to examine the cluster validity indices (CVIs) [63, 64]. There are internal CVIs and external indices which are commonly used in assessing the clustering functioning. The internal CVIs are prone to check cluster purity, while the external indices are used to validate the results based on a known accurate clustering outcome. Table 3 briefly summarizes the commonly used CVIs with their evaluation criteria.
Employing the listed methods, we test a different number of clusters on our data, ranging from two to 7 clusters using a R technique proposed by Montero and Vilar [68]. Tabular array 4 summarizes the clustering results. The consensus of two clusters is reached by fulfilling a majority of the CVI indices. The results testify 547 firms vest to Cluster 1 with 1072 firms in Cluster 2.
By analysing the extracted members in each cluster, nosotros examine the ii clusters by comparing their stock fourth dimension series patterns (come across Fig. 3). A weaker softening trend of stock price is observed in Cluster 1 amid COVID-nineteen outbreak. The stock prices quickly level off and regain an upward tilt after 23rd March. Thus, these firms seem to exist less sensitive to the COVID-nineteen pandemic. The stock prices in Cluster 2 testify a L-shaped movement along the sample period, whereby a steeper decline is observed with a slower charge per unit of recovery. The firms are more likely to exist sensitive to the COVID-19 pandemic. Comparing the centroids of the two clusters (see Fig. iv), nosotros find a K-shaped recovery where different sectors recover at different rates and magnitudes and the path of recovery continues to diverge.
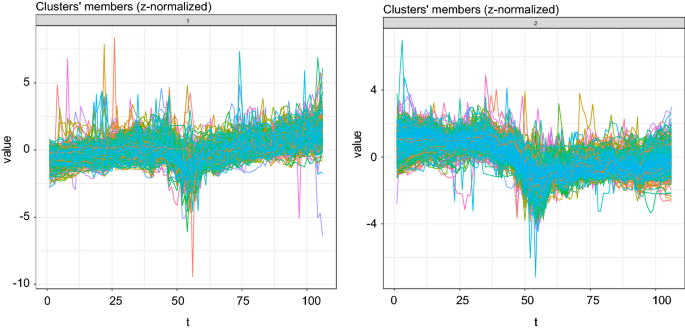
Members of Cluster 1 (left) and Cluster 2 (right)
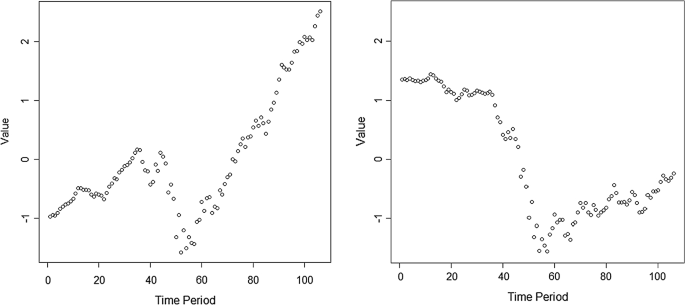
Centroid of Cluster 1 (left) and Cluster ii (correct)
To address the research query, the percentage of firms under the "Slow Recovery Cluster" and the "Better Recovery Cluster" is cross-tabulated with the level of digital transformation, with intensive and less intensive levels post-obit the OECD taxonomy of digital-intensity. The OECD taxonomy categorizes sectors into "high", "medium–high", "medium–low" and "depression" digital intensity depending on their relative position in the overall economies' ranking. Quartile rankings is used to measure how a sector has performed against all other sectors in terms of digital intensity. The rankings range from "Acme 25%" to "Lesser 25%". The tiptop 25% sectors are assigned to "high", followed by the 2nd highest 25% sectors denoted as "medium–high". Those with the lowest 25% digital intensity are labelled as "depression", and the second lowest 25% are named "medium–low". We assigned the sectors in the categories of "low", "medium–low" and "medium–high" into Group 2 the "less digital intensive" prepare, whereas the sectors of "high" digital intensity are categorized as "digital intensive" into Grouping one. This nomenclature is in line with OECD's methodology for implementation of the G20 roadmap for digitalisation, which compares sectors of operation that are among the top 25% of digital-intensive sectors versus non (Superlative Digital Intensive vs Less) [43].Transport equipment and telecommunications are members of the digital intensive sectors, whereas agricultural commodities and chemical products are amongst less digital intensive industries. Analysis is conducted to validate if the level of digital intensity is related with the two clusters, i.eastward., "Tedious Recovery Cluster" and "Ameliorate Recovery Cluster". The descriptive summary of the ho-hum recovery cluster and the better recovery cluster can be establish in Tabular array 5.
Consistent with expectation, a bigger portion of firms (47%) within the highly digitally transformed industries, such every bit telecommunications, Net retail and professional person services, falls under the "Better Recovery Cluster", as indicated in Table 5. 80% of the firms in the lower rung, including agriculture, fall in the "Boring Recovery Cluster". In addition, Pearson \(\chi^{2}\) statistic is used to examine the two-fashion associations with the number of firms in the cells (\(\chi^{two}\)(ane) = 128.51, p < 0.001). We observed that the top industries which take 50% companies or above fall under the "improve recovery cluster" including food retail (ninety%), Internet retail (77%), figurer communications (69%) and major telecommunication (50%). All these sectors belong to the "digital intensive" category except for nutrient retail. However, the potent performance of the food retail sector can be explained past the nature of the business. Equally the pandemic spreads beyond, the concern is expected to operate and go along to provide essential goods to households and communities 24-hour interval by day. However, the urge for nutrient retail to adopt digital transformation is clear because of increased online and mobile sales [69]. In comparing, the industries in the lower rung which has less than 20% of firms under the "better recovery cluster" include electric utilities (fourteen%), movies/amusement (xiv%), other transportation (xiv%), agronomical bolt/milling (12%), clothes/footwear retail (11%), construction materials (10%), water utilities (8%), real estate investment trusts (7%), real estate evolution (ii%) and media conglomerates (0%). All these sectors are of less digital intensity except for the sector of other transportation. However, the financial performance of this sector is largely affected past the need stupor acquired by COVID-19 crisis, excess supply, and significant oil toll decline and is less likely to be linked to its digital intensity. The results prove some directional support toward the relative touch of digital intensity. It indicates the degree of digital transformation (digital intensive, less digital intensive) is a relevant grouping variable which could be further tested in the Phase 2 assay.
Stage two Results
From the plotted pair of proxies– the NASDAQ alphabetize and the daily cumulative Twitter postings on COVID-19, negatively correlated motion of the two proxies is nowadays. Stock prices collapse when tweets about "COVID-xix" increase. Instead of testing correlation between proxy variables, based on the results in Phase 1, we adopt VAR model to examine the mutual causality human relationship between the daily cumulative Twitter postings on COVID-xix and stock price fluctuation under different levels of digital intensity [34, 70, 71]. Ane way is to examination the furnishings of posting trends on the stock price fluctuation for each respective grouping of companies. The other fashion is to examine for each group of firms if the stock toll fluctuation induces or reduces public concerns on COVID-nineteen. We use Granger causality to identify the leading relationship between the stock toll fluctuation and the daily cumulative twitter postings on COVID-19. We besides investigate the timing and length of the effects. The algorithm is implemented in R (Fig. 5).
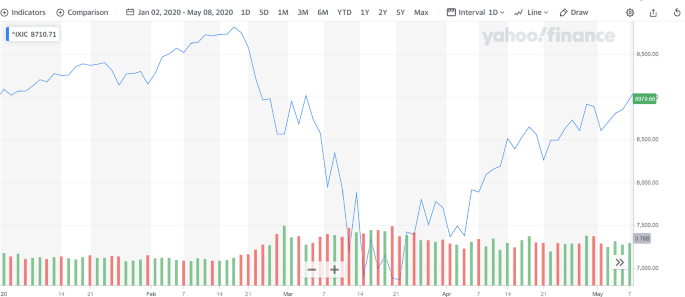
NASDAQ Composite (^IXIC) Charts
To ensure the validity of Granger-causality exam, we need to validate the stationarity of both fourth dimension series that enter the analysis because the non-stationarity of 1 or both time serial would cause spurious causality [72]. We employ Augmented Dickey–Fuller (ADF) Exam and Kwiatkowski–Phillips–Schmidt–Shin (KPSS) to cheque stationarity for both time series. The zip hypothesis of the old test is that the time series are non-stationary. The null hypothesis for the latter is stationarity to behave a cross-check. Tabular array half dozen presents the ADF and KPSS statistics for the daily cumulative Twitter postings on COVID-19 and the average daily stock price variation for each group of firms. The daily variation is the average of mean solar day-on-day price change of the firms in each group. The ADF and KPSS results show that the start-order difference eliminates stationarity for both time series. It besides determines the gild of integration and time series models [73].
$$Y_{t} { } = { }a_{0} { } + { }a_{ane} Y_{t - 1} { } + { } \cdots + { }a_{p} Y_{t - p} + { }b_{1} X_{t - 1} { } + { } \cdots + { }b_{p} X_{t - p} { } + { }u_{t}$$
(2)
$$X_{t} { } = { }c_{0} { } + { }c_{ane} X_{t - 1} { } + { } \cdots + { }c_{p} X_{t - p} + { }d_{1} Y_{t - 1} { } + { } \cdots + { }d_{p} Y_{t - p} { } + { }v_{t} { }$$
(iii)
Post-obit the Toda-Yamamoto (TY) procedure, Eq. (ii) and (three) are constructed. Y t denotes the average daily stock price variation in percentage. X t represents the daily cumulative Twitter postings on COVID-19. p denotes the lag gild, and a p , b p , c p , d p , are the coefficients of Y t-p and 10 t-p with the constant terms a 0 and c 0 . \(u_{t}\) and \(v_{t}\) represent the error terms in Eq. (2) and (3) respectively. The null hypothesis for Eq. (ii) is H 0 :b 1 =b two = … =b p= 0 with the alternative hypothesis H A : 'Not H 0 '. If H0 can be rejected, 10 is the Granger-cause of Y. Equation (3) tests Granger causality from the other direction—that is, H 0 :d 1 =d 2 = … =d p= 0 and H A : 'Not H 0 '. Similarly, if H0 could exist rejected, Y is the Granger-cause of 10. In another word, Eq. (2) is to see if the prior values of X together with Y's ain past values can predict Y better than solely using Y's own history. Equation (3) is to run into if the predictions of 10 can exist better fabricated on its own history values and Y'south past values than the predictions of X based on its ain history.
Table half-dozen shows the get-go gild differencing of X and Y eliminating the unit root, which determines the maximum order of integration as one, denoted I (1). Co-ordinate to the TY procedure, the VAR model uses the levels of the data without differencing. Another consideration for X and Y is to determine the appropriate lag length. Co-ordinate to the information criteria of Akaike Information Criterion (AIC), Hannan Quinn (HQ), Schwarz Criterion (SC) and Final Prediction Error (FPE), a lag of vi is deemed appropriate for the model. Toda and Yamamoto [73] point out the reward of the TY method is to save the cointegration exam and avoid pre-test bias. Additionally, we need to ensure no residual serial correlation in the specified VAR model. We conduct a Portmanteau test and observe Lag 6 removes residual serial autocorrelation.
The VAR model with Lag six is selected and 1 additional lag is added to each equation as the maximum lodge of integration—I(i). Then, we construct the augmented VAR model for Eq. (2) and Eq. (3). A Wald exam is used to test the Granger causality hypothesis whether the coefficients of the first vi lagged values of 10 in Eq. (2) and the coefficients of the start half-dozen lagged values of Y in Eq. (3) are zip. The coefficient of the seventh lag is not included because information technology is the additional lagged value to fix the asymptotics and ensure Wald test statistics of asymptotical chi-square distribution. When the naught hypothesis of Wald test is rejected, a Granger causality is implied. The results are presented in Table 7.
Table 7 shows that Twitter postings on COVID-19 Granger cause the stock cost changes of firms in Group 1 and Group ii. The stock price fluctuation in Groups 1 and two too imposes an bear on on cumulative Twitter postings on COVID-19. These results show that the fluctuation of stock prices would trigger a swing in market sentiment and vice versa. Every bit a further investigation footstep, we test the magnitudes and the directions of these impacts using the augmented VAR models with the results in Tables 8 and 9.
As nosotros are interested to differentiate the impact of Twitter postings on stock cost fluctuation amongst the firms of different levels of digital intensity, a detailed investigation of Eq. (2) is made on two groups of firms—one digital intensive (Group one), the other 1 less digital intensive (Group ii). Consistent with our prediction, the cumulative Twitter postings are negatively related to the stock toll fluctuation of firms in Group ane, lagged by two days. A two-menses lag is found with stronger negative bear upon of Twitter postings on stock price in Group 2. This indicates cumulative Twitter postings would trigger stronger negative changes in stock prices of firms in Group 2 than those in Group i. It is axiomatic that digital intensity of firms mitigates the negative consequence of market sentiments considering of largescale unanticipated crunch on stock marketplace performance. When stocks prices collapse amid COVID-19 pandemic, firms with high degree of digital intensity have out-performed the others.
The results of Eq. (iii) show the bear upon of stock cost variation on cumulative Twitter postings for firms in Group 1 and those in Group ii. As seen from Table 9, stock price changes crusade swings of cumulative Twitter postings in a negative direction for Group 1 and Group 2, lagged by ane flow. In terms of magnitude of the touch, stock price increase will atomic number 82 to a deeper decline of cumulative Twitter postings in Group 1. The impact on cumulative Twitter postings is stronger for the firms in Grouping 1 than for those in Group two.
Word
Contributions to Noesis, Methodology and Practice
This research has set up out to examine how market place sentiments touch the stock market functioning nether COVID-xix. Market sentiments are modelled with cumulative social media postings on COVID-xix, which reflect the overall consensus about the market equally a whole. Performance is operationalised by the stock price differences of firms within the sectors. All sectors under investigation are organized co-ordinate to OECD Digital-intensity Framework.
Assay is conducted in two phases. Stage one establishes a two-group model on how stock prices accommodate to the sudden emergence of the COVID-xix pandemic. Stock prices of a bulk of firms across the most digitally advanced sectors have remained resilient when an unexpected crisis puts the market to test, while sectors that lag behind are among the most negatively impacted.
Phase 2 confirms that cumulative Twitter postings on COVID-xix are predictive of stock performance. There is further evidence to demonstrate that the sectoral digital intensity of firms tin can mitigate the adverse impact of market sentiments induced past largescale unanticipated events. As stock prices dipped amid the pandemic, the digital "have-mores" out-performed the remainder and recovered quickly subsequently the crash. Findings in Stage ii also propose that stock toll changes tin in plow influence market sentiments. That is, the market has responded more than favourably towards the most digitally advanced firms and sectors and more negatively towards the laggards under crisis.
Governments worldwide have imposed lockdowns in the cities to slowdown the magnitude and speed at which the pandemic is developing. Such lockdowns have led to a near complete standstill of much corporeal economic activities. All the same, certain business concern trades and consumer purchases have not but connected online, but really grown considerably. Demand for digital services and portable computing devices take risen as people switch to remote working and learning. Many are envisioning the emergence of a mail service-COVID-19 'new normal', where there will be a strong prominence of digitalisation. In view of these developments, the market place may have given eminence to and greater conviction in firms and sectors that are nigh digitally advanced as they are in a better position to sustain operations not only amidst the pandemic, but also to leverage the total potential in the mail-COVID-19 'new normal'. Thus, we observe a pronounced gap between the digital "haves" and "have-mores" in their stock market performance.
This study contributes to understanding most how digital intensity moderates the effect of market sentiments on firms' stock prices, particularly due to largescale unanticipated events such every bit a pandemic. Researchers admit the importance of alignment between a business firm's concern and digital strategies [74]. Information engineering (IT) strategy and technological investments have been found to influence profitability and the marketplace value of the firm [75]. While extant inquiry has examined the utilise of technology and organizational performance [76], this written report is among the first few to demonstrate how sectors and companies on the digital borderland can requite ascent to disproportionate gains in the stock market. While past research suggests that the stock market reacts more positively to announcements related to digital investments, such as appointments of chief digital officers [77] or engaging in eastward-commerce [78], our findings focus on sectoral digitalisation as the potential mitigating circumstances when bad news occurs.
The findings besides suggest that people do have recent stock prices into consideration when posting online about COVID-19, and such posting behaviours will in turn drive future stock price changes. Yet, the magnitude of such effect depends on the level of sectoral digital intensity.
In terms of methodology, while the majority of conventional enquiry have studied the impact of largescale unexpected incidents on stocks using intervention analysis [32] or event studies [33], this study demonstrated the feasibility of using cumulative social media postings on negative events to mensurate marketplace sentiments, especially on largescale unanticipated events that can touch the stock prices of firms across sectors. This presents researchers with an culling to quantify market sentiments and expand the inquiry arsenal to comprise social media trends, which tin can offer college explanatory ability.
Limitations and Time to come Inquiry
This inquiry has some limitations which offering opportunities for futurity investigation. Firstly, the study looks into how sectoral digital intensity mitigates the impact of negative market sentiments on the stock market, focusing on the catamenia before, during and after the March 2020 COVID-nineteen stock market place crash. It is possible that there is already a gap between the digital 'Haves' and 'Have-mores' in their stock performance before the crunch. Past research has confirmed that the stock market reacts positively to firms that comprehend digital initiatives, such as appointments of newly created chief digital or data officer positions [77] or engaging in eastward-commerce [78]. Future studies can look into the long-run stock price performance of firms with effective digital strategies.
Secondly, afterward the March 2020 COVID-19 stock marketplace crash, many of the major stock indices take regained much of their lost territory since early on Apr, and such recovery and so far has been fuelled by aggressive stimulus packages rolled out by governments to boost the economy. The resuming of business activities and trade is also underpinning market optimism and rebound. The misreckoning furnishings of authorities intervention and support are hard to capture within the current model. Future enquiry tin examine how such authorities intervention restores public conviction and accelerates the market rebound. Moreover, this research adopts a batch processing methodology. Hereafter research can look into real-time prediction of stock performance using social media streaming [47].
Third, the literature on sectoral digital intensity suggests a large and widening divide amid firms within each sector. We did not capture the within sector relative digital intensity in the current written report. Futurity inquiry should compare the digital "haves" and "have mores" within each sector.
Lastly, while the speed of innovation is accelerating economy-wise, not all firms inside the same sectors are similarly equipped to answer to new challenges and tap into new opportunities. This research has not looked into the possible causes of the widening divide between sectors in digital intensity. With the entry of new digital start-ups and technology firms and the emergence of new marketplace segments, sectoral boundaries are blurring. Future research may aim to uncover how the diverse conditions lead to an increasing separate betwixt the "haves" and the "have-mores". A longitudinal report to identify the changes in digital capabilities within each sector can aid reveal the procedure of such changes.
References
-
Mazur M, Dang Thou, Vega M (2021) COVID-xix and the March 2020 stock market crash: evidence from S&P1500. Financ Res Lett 38:1090. https://doi.org/10.1016/j.frl.2020.101690
-
Bollen J, Mao H, Zeng X (2011) Twitter mood predicts the stock market. J Comput Sci 2(1):1–8. https://doi.org/10.1016/j.jocs.2010.12.007
-
Huang X, Kuijpers D, Li Fifty, Sha S (2020) Xia C (2020) How Chinese consumers are irresolute shopping habits in response to COVID-19, McKinsey & Company. McKinsey & Company Accessed half-dozen:2020
-
Sheth J (2020) Impact of Covid-19 on consumer behavior: Will the old habits return or dice? J Omnibus Res 117:280–283. https://doi.org/10.1016/j.jbusres.2020.05.059
-
Kshetri Due north (2020) COVID-nineteen Meets Big Tech. Computer 53(08):10–13. https://doi.org/10.1109/MC.2020.2996698
-
Saxena N, Gupta P, Raman R, Rathore AS (2020) Role of data scientific discipline in managing COVID-nineteen pandemic. Indian Chem Eng 62(4):385–395. https://doi.org/10.1080/00194506.2020.1855085
-
Sliwoski Grand, Kothiwale S, Meiler J, Lowe EW Jr (2013) Computational methods in drug discovery. Pharmacol Rev 66(1):334–395. https://doi.org/10.1124/pr.112.007336
-
Wong ZSY, Zhou J, Zhang Q (2019) Bogus Intelligence for infectious disease Big Information Analytics. Infect Dis Health 24(i):44–48. https://doi.org/10.1016/j.idh.2018.10.002
-
Mohamadou Y, Halidou A, Kapen PT (2020) A review of mathematical modeling, artificial intelligence and datasets used in the study, prediction and management of COVID-nineteen. Appl Intell 50(eleven):3913–3925. https://doi.org/ten.1007/s10489-020-01770-9
-
Wang P, Lu J-A, Jin Y, Zhu M, Wang L, Chen S (2020) Statistical and network analysis of 1212 COVID-nineteen patients in Henan, China. Int J Infect Dis 95:391–398. https://doi.org/x.1016/j.ijid.2020.04.051
-
Livadiotis G (2020) Statistical assay of the impact of ecology temperature on the exponential growth rate of cases infected by COVID-19. PLoS ONE 15(5):e0233875–e0233875. https://doi.org/x.1371/journal.pone.0233875
-
Menebo MM (2020) Temperature and precipitation associate with Covid-19 new daily cases: A correlation study between weather condition and Covid-19 pandemic in Oslo. Norway Sci Total Environ 737:139659. https://doi.org/10.1016/j.scitotenv.2020.139659
-
Bragazzi NL, Dai H, Damiani G, Behzadifar One thousand, Martini Grand, Wu J (2020) How Big Data and Bogus Intelligence Tin Assistance Better Manage the COVID-19 Pandemic. Int J Environ Res Public Health 17(nine):3176. https://doi.org/ten.3390/ijerph17093176
-
Calvino F, Criscuolo C, Marcolin L, Squicciarini M (2018) A taxonomy of digital intensive sectors. OECD Science, Technology and Industry Working Papers. https://doi.org/x.1787/f404736a-en
-
Sul HK, Dennis AR, Yuan 50 (2017) Trading on Twitter: using social media sentiment to predict stock returns. Decis Sci 48(3):454–488. https://doi.org/10.1111/deci.12229
-
Sprenger TO, Tumasjan A, Sandner PG, Welpe IM (2014) Tweets and trades: the data content of stock microblogs. Eur Financ Manag 20(v):926–957. https://doi.org/10.1111/j.1468-036X.2013.12007.ten
-
De Bondt WFM, Thaler R (1985) Does the stock market place overreact? J Financ 40(3):793–805. https://doi.org/ten.1111/j.1540-6261.1985.tb05004.x
-
Nofsinger JR (2005) Social mood and financial economics. J Behav Financ 6(3):144–160. https://doi.org/10.1207/s15427579jpfm0603_4
-
Barrett WB, Heuson AJ, Kolb RW, Schropp GH (1987) The aligning of stock prices to completely unanticipated events. Financ Rev 22(4):345–354. https://doi.org/10.1111/j.1540-6288.1987.tb01258.x
-
Shelor RM, Anderson DC, Cross ML (1992) Gaining from loss: property-liability insurer stock values in the aftermath of the 1989 California convulsion. J Chance Insur 59(3):476–488. https://doi.org/10.2307/253059
-
Lee G-J, Lu S-L, Shih Y (2018) Contagion issue of natural disaster and financial crunch events on international stock markets. J Hazard Financ Manag eleven(ii):16. https://doi.org/ten.3390/jrfm11020016
-
Cam MA, Ramiah V (2014) The influence of systematic risk factors and econometric adjustments in catastrophic effect studies. Rev Quant Finan Acc 42(2):171–189. https://doi.org/10.1007/s11156-012-0338-iv
-
Pompian M (2012) Behavioral finance and wealth direction: how to build investment strategies that business relationship for investor biases. Wiley
-
Shehzad K, Liu X, Kazouz H (2020) COVID-19's disasters are perilous than Global Financial crunch: a rumour or fact? Financ Res Lett 36:101669. https://doi.org/10.1016/j.frl.2020.101669
-
McTier BC, Tse Y, Wald JK (2013) Do Stock Markets Catch the Flu? J Financ Quant Anal 48(iii):979–1000. https://doi.org/ten.1017/S0022109013000239
-
Li Y (2020) Market reactions to past virus scares show stocks may have more to lose. CNBC. https://www.cnbc.com/2020/01/28/market place-reactions-to-major-virus-scares-show-stocks-have-more-to-lose.html
-
Leduc South, Liu Z (2020) The Uncertainty Channel of the Coronavirus. FRBSF. Accessed 30 March 2020
-
Tooze A (2020) The crunch has brought the economy to a near halt, and left millions of people out of piece of work. But thanks to intervention on an unprecedented scale, a full-scale meltdown has been averted—for at present. The Guardian. Accessed 2020 2020
-
Georgieva Yard (2020) International monetary fund managing manager Kristalina Georgieva'southward statement following a G20 ministerial phone call on the coronavirus emergency. Imf. Accessed 23 March 2020 2020
-
Banerji K (2020) Why did stock markets rebound from Covid in tape fourth dimension? Here are v reasons. Wall Street J. Accessed fifteen September 2020
-
Winck B (2020) Cries for more than stimulus are overblown and stock investors should stop throwing 'tantrums' about it, says a Wall Street master strategist. Business Insider. Accessed 2020
-
Box GEP, Tiao GC (1975) Intervention analysis with applications to economic and environmental problems. J Am Stat Assoc 70(349):lxx–79. https://doi.org/ten.1080/01621459.1975.10480264
-
MacKinlay AC (1997) Event studies in economics and finance. J Econ Lit 35(1):13–39
-
Liu Y, Peng Thou, Hu L, Dong J, Zhang Q (2019) Using Google Trends and Baidu Index to analyze the impacts of disaster events on visitor stock toll. Ind Manag Data Syst 120(two):350–365. https://doi.org/10.1108/IMDS-03-2019-0190
-
Ding D, Guan C, Chan CML, Liu W (2020) Building stock market resilience through digital transformation: using Google trends to analyze the impact of COVID-19 pandemic. Front Bus Res China 14:21. https://doi.org/10.1186/s11782-020-00089-z
-
Baker G, Wurgler J (2007) Investor Sentiment in the Stock Market place. J Econ Perspect 21(2):129–152. https://doi.org/10.2139/ssrn.962706
-
boyd dm, Ellison NB, (2007) Social network sites: definition, history, and scholarship. J Comput-Mediat Comm 13(1):210–230. https://doi.org/10.1111/j.1083-6101.2007.00393.x
-
Eastward Chiliad, K 1000 Widespread worry and the stock market place. In: ICWSM 2010 - Proceedings of the quaternary International AAAI Conference on Weblogs and Social Media, Washington, DC, 2010. pp 58–65
-
Smailović J, GrÄŤar Grand, LavraÄŤ N, Ĺ˝nidaršiÄŤ M (2014) Stream-based active learning for sentiment analysis in the fiscal domain. Inf Sci 285:181–203. https://doi.org/ten.1016/j.ins.2014.04.034
-
Saini A, Sharma A (2019) Predicting the unpredictable: an application of machine learning algorithms in Indian stock market. Ann Information Sci. https://doi.org/10.1007/s40745-019-00230-7
-
Baig A, Hall B, Jenkins P, Lamarre Due east (2020) McCarthy B (2020) The COVID-19 recovery will exist digital: A plan for the first 90 days. McKinsey & Company Accessed 14:2020
-
Paunov C, Planes-Satorra S (2019) How are digital technologies changing innovation?: Evidence from agronomics, the automotive industry and retail. OECD Publishing, OECD Science. https://doi.org/10.1787/67bbcafe-en
-
OECD (2018) Towards the implementation of the G20 roadmap for digitalisation: skills, business concern dynamics and competition. Written report prepared at the request of the 2017 G20 High german Presidency
-
Neu WA, Brownish SW (2005) Forming successful business organisation-to-business services in goods-dominant firms. J Serv Res 8(1):3–17. https://doi.org/10.1177/1094670505276619
-
Noventum Service Management (2016) Manufacturers' avant-garde services: IoT every bit the key to profitability and growth. White Newspaper. Accessed 2016
-
Coreynen Due west, Matthyssens P, Bockhaven WV (2017) Boosting servitization through digitization: Pathways and dynamic resource configurations for manufacturers. Ind Market Manag 60:42–53. https://doi.org/10.1016/j.indmarman.2016.04.012
-
Tien JM (2017) Net of Things, Real-Fourth dimension Decision Making, and Artificial Intelligence. Ann Information Sci 4(2):149–178. https://doi.org/10.1007/s40745-017-0112-five
-
Okuda A, Karazhanova A (2020) Digital Resilience Against COVID-19. United Nation ESCAP. Accessed 31 March 2020
-
Blackburn S, LaBerge L, O'Toole C (2020) Schneider J (2020) Digital strategy in a time of crisis. McKinsey & Company Accessed 22:2020
-
Sard´a-Espinosa A (2018) Comparing Time-Series Clustering Algorithms in R Using the dtwclust Packet
-
Paparrizos J, Gravano L (2017) Fast and accurate time-series clustering. Acm T Database Syst 42(2):8. https://doi.org/10.1145/3044711
-
Shi Y, Tian YJ, Kou G, Peng Y, Li JP (2011) Optimization based information mining: theory and applications. Springer
-
Bordino I, Battiston South, Caldarelli Yard, Cristelli M, Ukkonen A, Weber I (2012) Web search queries tin can predict stock market place volumes. PLoS ONE 7(7):e40014. https://doi.org/10.1371/journal.pone.0040014
-
Weng B, Lu L, Wang Ten, Megahed FM, Martinez West (2018) Predicting brusk-term stock prices using ensemble methods and online information sources. Adept Syst Appl 112:258–273. https://doi.org/x.1016/j.eswa.2018.06.016
-
Analytics T (2020) Covid xix—Twitter evolution. Tweet Folder. Accessed 8 May 2020 2020
-
Aghabozorgi S, Seyed Shirkhorshidi A, Ying Wah T (2015) Fourth dimension-series clustering – A decade review. Inf Syst 53:xvi–38. https://doi.org/10.1016/j.is.2015.04.007
-
Olson DL, Shi Y (2007) Introduction to business data mining. McGraw-Hill/Irwin
-
Liao TW (2005) Clustering of time series data-a survey. Pattern Recognit 38(eleven):1857–1874. https://doi.org/ten.1016/j.patcog.2005.01.025
-
Golay X, Kollias Southward, Stoll G, Meier D, Valavanis A, Boesiger P (1998) A new correlation-based fuzzy logic clustering algorithm for fMRI. Magn Reson Med 40(2):249–260. https://doi.org/x.1002/mrm.1910400211
-
Hastie T, Tibshirani R, Friedman J (2009) The elements of statistical learning. Springer
-
Giorgino T (2009) Computing and visualizing dynamic fourth dimension warping alignments in R: the dtw package. J Stat Softw 31(7):1–24
-
Giorgino T (2009) Computing and visualizing dynamic time warping alignments in R: the dtw package. J Stat Softw 31(i07):1–24
-
Arbelaitz O, Gurrutxaga I, Muguerza J, PĂ©rez JM, Perona I (2013) An extensive comparative study of cluster validity indices. Pattern Recognit 46(ane):243–256. https://doi.org/10.1016/j.patcog.2012.07.021
-
Lei Y, Bezdek JC, Chan J, Vinh NX, Romano S, Bailey J (2017) Extending information-theoretic validity indices for fuzzy clustering. IEEE Trans Fuzzy Syst 25(4):1013–1018. https://doi.org/10.1109/TFUZZ.2016.2584644
-
Rousseeuw PJ (1987) silhouettes: a graphical help to the interpretation and validation of cluster analysis. J Comput Appl Math 20:53–65. https://doi.org/10.1016/0377-0427(87)90125-seven
-
Saitta S, Raphael B, Smith IFC (eds) (2007) A Bounded Index for Cluster Validity, vol 4571. Machine Learning and Data Mining in Pattern Recognition. MLDM 2007. Lecture Notes in Computer Scientific discipline. Springer
-
Kim M, Ramakrishna RS (2005) New indices for cluster validity assessment. Pattern Recogn Lett 26:2353–2363
-
Montero P, Vilar JA (2014) TSclust: An R Parcel for Time Series Clustering. J Stat Softw 62(one):1–43
-
Markenson S (2020) Grocery's Ecommerce Evolution during COVID-19. Food Marketing Found. https://world wide web.fmi.org/blog/view/fmi-blog/2020/06/23/grocery-s-ecommerce-evolution-during-covid-19
-
Tetlock PC (2007) Giving content to investor sentiment: the role of media in the stock market. J Financ 62(3):1139–1168. https://doi.org/ten.1111/j.1540-6261.2007.01232.ten
-
Deng S, Huang ZJ, Sinha AP, Zhao H (2018) The interaction betwixt microblog sentiment and stock render: an empirical examination. MIS quart 42(3):895–918
-
He Z, Maekawa K (2001) On spurious Granger causality. Econ Lett 73(iii):307–313. https://doi.org/ten.1016/S0165-1765(01)00498-0
-
Toda HY, Yamamoto T (1995) Statistical inference in vector autoregressions with possibly integrated processes. J Econom 66(1–2):225–250. https://doi.org/x.1016/0304-4076(94)01616-viii
-
Sabherwal R, Sabherwal S, Havakhor T, Steelman Z (2019) How does strategic alignment affect house performance? the roles of information technology investment and environmental uncertainty. MIS Q 43(ii):453–474. https://doi.org/10.25300/misq/2019/13626
-
Mithas Due south, Rust RT (2016) How data engineering science strategy and investments influence firm performance: theorize and empirical prove. MIS Q forty(ane):223–245. https://doi.org/10.25300/misq/2016/40.i.10
-
Hanelt A, Firk S, Hildebrandt B, Kolbe LM (2021) Digital Thou&A, digital innovation, and firm performance: an empirical investigation. Eur J Inf Syst thirty(i):3–26. https://doi.org/10.1080/0960085X.2020.1747365
-
Hendricks KB, Singhal VR (2001) The Long-Run Stock Price Performance of Firms with Constructive TQM Programs. Manag Sci 47(iii):359–368. https://doi.org/ten.1287/mnsc.47.3.359.9773
-
Subramani M, Walden E (2001) The Impact of East-Commerce Announcements on the Market Value of Firms. Inf Syst Res 12(ii):135–154. https://doi.org/10.1287/isre.12.2.135.9698
Author information
Affiliations
Corresponding author
Ethics declarations
Conflicts of interest
The writer declared they have no conflict of interest.
Additional information
Publisher'south Note
Springer Nature remains neutral with regard to jurisdictional claims in published maps and institutional affiliations.
Rights and permissions
Well-nigh this commodity
Cite this article
Guan, C., Liu, Westward. & Cheng, J.YC. Using Social Media to Predict the Stock Market Crash and Rebound among the Pandemic: The Digital 'Haves' and 'Have-mores'. Ann. Information. Sci. nine, 5–31 (2022). https://doi.org/ten.1007/s40745-021-00353-w
-
Received:
-
Revised:
-
Accepted:
-
Published:
-
Issue Appointment:
-
DOI : https://doi.org/10.1007/s40745-021-00353-westward
Keywords
- Stock market
- COVID-19
- Social media
- Digital intensity
0 Response to "Nyse Article About Against Social Media"
Post a Comment